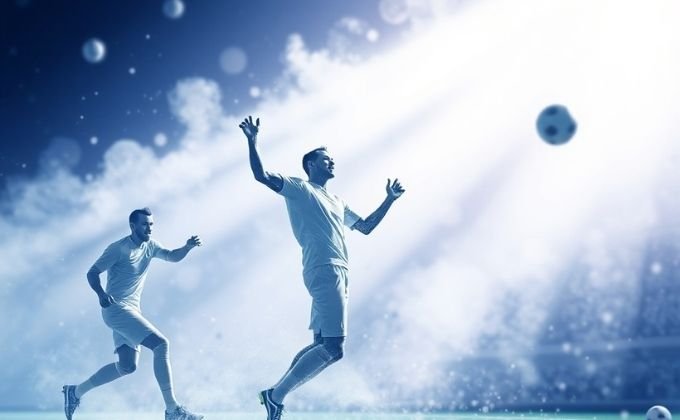
In recent years, artificial intelligence (AI) has revolutionized several industries, and sports prediction is no exception. AI sports predictions have become an invaluable tool for enthusiasts, analysts, and even professional sports teams looking to gain an edge in their strategies. Companies like Playstat are harnessing AI to offer predictions that are not only more accurate but also faster and more comprehensive than traditional methods. These AI-driven predictions use complex algorithms to analyze massive datasets, helping users make informed decisions based on patterns, trends, and statistics. In this article, we will explore how AI sports predictions work and the science behind the algorithms driving them.
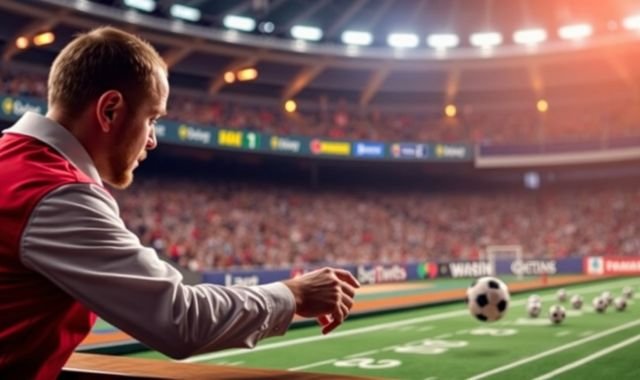
Understanding AI Sports Predictions
AI sports predictions are built on the foundation of machine learning, which is a subset of AI that enables computers to learn and make decisions without human intervention. By processing and analyzing large amounts of historical data, AI systems can identify patterns, correlations, and anomalies that humans might miss. These systems continuously improve by learning from new data and refining their models, making predictions more accurate over time.
Playstat, a leader in AI sports predictions, employs cutting-edge machine learning algorithms to forecast outcomes for various sports, including football, basketball, cricket, and more. Their technology is capable of evaluating countless factors that influence the outcome of a game, such as player performance, team dynamics, weather conditions, and historical trends.
The Role of Data in AI Sports Predictions
At the heart of AI sports predictions lies data. The more comprehensive and accurate the data, the better the prediction model. Data sources include a variety of metrics such as player statistics (goals scored, assists, turnovers, etc.), team performance, injury reports, and even external factors like weather or crowd attendance. By inputting this information into a machine learning model, AI can process the data at a scale and speed that humans cannot replicate.
The quality of data is paramount in AI predictions. If the data is incomplete, outdated, or biased, the algorithm’s predictions can be flawed. This is why companies like Playstat invest heavily in gathering high-quality, real-time data from multiple reliable sources to ensure that their predictions are as accurate as possible.
The Science of Machine Learning Algorithms
Machine learning algorithms are the backbone of AI sports predictions. These algorithms are designed to recognize patterns within data and use them to make predictions. There are several types of machine learning algorithms used in sports prediction, including:
1. Supervised Learning
Supervised learning involves training a model on a labeled dataset, where the outcomes (such as match results) are already known. The algorithm then learns to associate certain features of the data (like player statistics, team history, etc.) with the corresponding outcomes. Over time, the model gets better at predicting outcomes based on the patterns it has learned.
For example, Playstat might use supervised learning to predict the winner of a football match by analyzing past matches and the performance of teams under similar conditions.
2. Unsupervised Learning
In unsupervised learning, the algorithm is provided with data that does not have labeled outcomes. The system must identify inherent patterns and structures in the data on its own. This type of learning is used to find hidden relationships or clusters of data points that might not be immediately obvious.
Unsupervised learning can be particularly useful for discovering trends or anomalies that could affect sports outcomes, such as changes in team dynamics, emerging player performance, or unexpected shifts in weather conditions.
3. Reinforcement Learning
Reinforcement learning involves training algorithms to make decisions based on feedback. The system learns by interacting with its environment and receiving rewards or penalties based on its actions. Over time, it learns the best strategy to maximize the reward. This type of learning can be applied to sports by simulating match conditions and evaluating the strategies that lead to successful outcomes.
4. Neural Networks
Neural networks, inspired by the human brain, are highly complex algorithms that can model complex relationships within data. These networks consist of layers of interconnected nodes, each performing mathematical calculations. Neural networks are particularly useful in recognizing non-linear patterns in large datasets, making them ideal for sports predictions where multiple variables interact in complex ways.
5. Ensemble Methods
Ensemble methods combine the predictions of several different models to improve accuracy. By aggregating the strengths of different algorithms, ensemble methods can reduce the risk of overfitting (when a model is too closely aligned with training data) and enhance the overall prediction reliability.
Playstat, for instance, may use ensemble methods to combine different algorithms and provide users with more accurate sports predictions.
The Challenges of AI Sports Predictions
While AI sports predictions have made significant strides, they are not without their challenges. One of the primary hurdles is the inherent unpredictability of sports. Unlike many other domains where variables are more controlled, sports outcomes can be influenced by a variety of factors, including injuries, referee decisions, and even luck. AI algorithms can account for a large number of variables, but they cannot predict every possible outcome.
Another challenge is data availability and accuracy. Inaccurate or incomplete data can significantly impact the reliability of predictions. This is why companies like Playstat ensure they have access to high-quality, real-time data feeds to power their AI systems.
The Future of AI Sports Predictions
As AI continues to evolve, so too will the accuracy and sophistication of sports predictions. In the near future, AI systems will likely incorporate more advanced techniques such as natural language processing (NLP) to analyze media reports, fan sentiment, and social media posts. Additionally, AI may become even better at factoring in real-time events, such as sudden injuries or changes in weather conditions, to adjust predictions during a game.
Moreover, as AI models become more personalized, fans and bettors will be able to receive tailored predictions based on their preferences and past behaviors. The integration of AI in sports prediction is set to transform how we view and engage with sports, making it more data-driven and insightful.
Conclusion
AI sports predictions represent a convergence of technology and athletics, offering unparalleled insights into the outcomes of games. Powered by machine learning algorithms, companies like Playstat are at the forefront of this innovation, providing accurate, real-time predictions across a wide range of sports. While challenges remain, the future of AI in sports prediction looks promising, with improvements in data accuracy, algorithm sophistication, and real-time analytics. As these technologies evolve, AI sports predictions will continue to reshape how fans, analysts, and professionals approach the game.
For more information or to get started with Playstat’s AI sports prediction services, you can reach out via email at marketing@playstat.com.